Synthetic Data Is a Dangerous Teacher
Synthetic Data Is a Dangerous Teacher
Synthetic data, which is artificially created data rather than being generated by real-world events, is increasingly being used in...
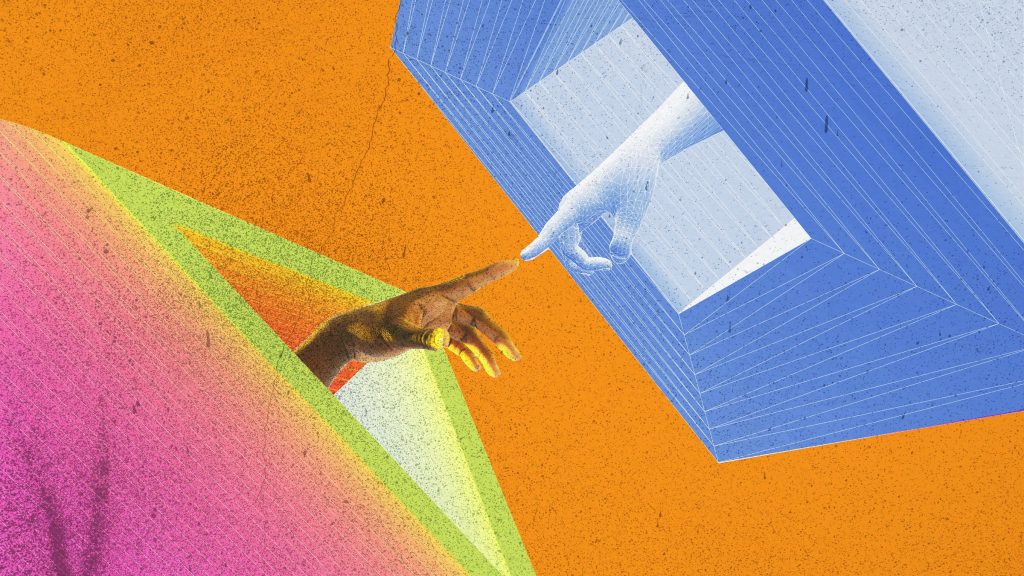
Synthetic Data Is a Dangerous Teacher
Synthetic data, which is artificially created data rather than being generated by real-world events, is increasingly being used in various industries for training machine learning models. However, relying solely on synthetic data can be a dangerous practice.
One of the main drawbacks of synthetic data is that it may not accurately reflect the complexities and nuances of the real-world data it is supposed to represent. This can lead to biased or inaccurate predictions when the trained model is deployed.
Moreover, synthetic data can inadvertently introduce harmful biases into the model, perpetuating discrimination and inequality. For example, if the synthetic data used to train a facial recognition system is predominantly of one ethnicity, the model may perform poorly on individuals from other ethnicities.
Another issue with synthetic data is its limited variability. Real-world data is constantly changing and evolving, whereas synthetic data is static and may not capture all the possible scenarios and edge cases that the model needs to handle.
Furthermore, using synthetic data exclusively may hinder the model’s ability to generalize well to unseen data. This can be particularly problematic in high-stakes applications such as healthcare or finance, where even slight errors can have severe consequences.
It is important for data scientists and machine learning practitioners to supplement synthetic data with real-world data to ensure the robustness and reliability of their models. This hybrid approach can help mitigate the risks associated with relying solely on synthetic data.
In conclusion, while synthetic data can be a useful tool for training machine learning models, it should be used judiciously and in conjunction with real-world data to avoid the pitfalls of biased predictions, limited variability, and poor generalization.